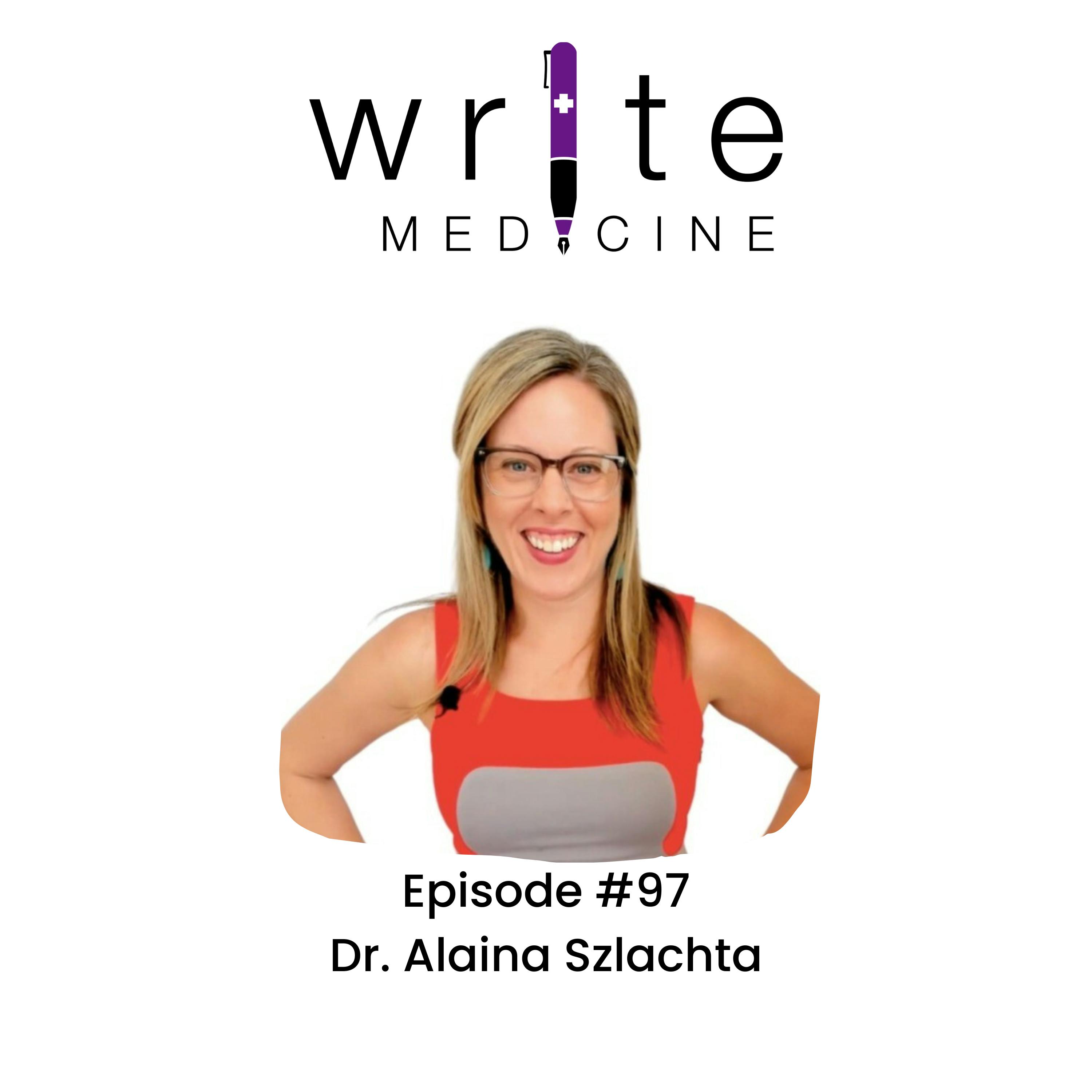
Are you curious about how the art of data collection and analysis can transform the impact of continuing education in the health professions? What if you could easily prove that learning activities drive tangible outcomes?
Today's guest is Dr. Alaina Szlachta, a Learning Architect who improves the results of personal and professional development programs through data enablement. She joins us to unravel the complexities of effective education activity and program evaluation.
In a world increasingly driven by data and evidence, understanding the outcomes and impact of educational programs is crucial, but learning professionals in many sectors often struggle to effectively evaluate education activity and program impact. And without proof of outcomes, it's difficult to demonstrate value and make data-driven decisions.
Alaina outlines the crucial checkboxes for successful evaluation and highlights the art and science of strategically aligning evaluation variables to forge a persuasive chain of evidence. We also tackle the sometimes daunting task of identifying behavioral change indicators and how to build "indicator muscle."
In this episode, you'll hear how to:
Tune in to hear how to build a rock-solid evaluation process in under an hour.
Email: alaina@bydesigndevelopmentsolutions.com
By Design Development Solutions
Don’t forget to subscribe to the Write Medicine podcast for more valuable insights on continuing medical education content for health professionals. Click the Follow button and subscribe on your favorite platform.
[0:05] Welcome to Write Medicine, where we explore best practices in creating continuing education content for health professionals.
I'm Alex Howson and I'm on a mission to share expert insights and field perspectives on topics like adult learning, content creation techniques, effective formats and trends in healthcare that influence the type of continuing education content that we create.
Bright Medicine is the premier podcast for CME CPD professionals like you, wherever you are in the content creation process. Join us.
[0:39] Music.
[0:47] At the end of the day, what all those models have in common is that they're essentially and eventually looking at did we make changes in people's thinking, in their knowledge base, and of course in critical actions and behaviors that we wanted them to do with their newly acquired knowledge.
[1:05] Music.
[1:13] Are you curious about how the art of data collection and analysis can transform the impact of continuing education in the health professions?
What if you could easily prove that learning activities drive tangible outcomes?
Today's guest is Dr. Alaina Szlachta, a learning architect who improves the results of personal and professional development programs through data enablement.
She hosts a community of practice, Measurement Made Easy, to explore simpler solutions for showing program outcomes and impact and is writing a book on measurement and evaluation on a shoestring, written to make measurement and evaluation easier and more accessible.
Elena joins us in episode 97 to unravel the complexities of effective education activity and program evaluation.
In a world increasingly driven by data and evidence, understanding the outcomes and impact of educational programs is crucial, but learning professionals in many sectors often struggle to effectively evaluate education activity and program impact.
And without proof of impact or outcomes, it's difficult to demonstrate value and make data-driven decisions.
[2:26] Alaina outlines the crucial checkboxes for successful evaluation and highlights the art and science of strategically aligning evaluation variables to forge a persuasive chain of evidence.
We also tackle the sometimes daunting task of identifying behavioral change indicators and how to build indicator muscle.
In this episode, you'll hear how to develop a simple framework for aligning learning outcomes, identify indicators that reliably track performance improvement, and leverage AI to efficiently create assessments tailored to your needs.
Tune in to hear how to build a rock-solid evaluation process in under an hour.
[3:08] Music.
[3:17] Alright, here we are. I would love for you to introduce yourself and tell us a little bit about who you are and the work that you do. Well, Alex, I'm so excited to be here.
You're my first podcast conversation of 2024.
You've probably had many. I know your podcast is infinitely famous, but I'm delighted to be here.
My name is Dr. Alaina Szlachta What I do for a living after having had many careers leading up to today, I won't bore you with all the details.
But present day, I am a consultant. I have a practice where I help people collect good data to show the outcomes and impact of their programs.
Mainly the people that I serve are folks in the nonprofit world, interestingly.
I'll tell you more about that. And of course, folks who do education, learning, and development.
And at the end of the day, the name of the game is making it easy to collect data because, my gosh, you can easily get lost in the weeds in the world of data, analytics, enablement, collection analysis and all of the things.
So my goal is to make all of that easier.
[4:24] Yeah, I love that you that you shared that. And you mentioned enablement.
So I definitely want to come back to that because that is a term that is actually going to be floated in one of the plenary sessions at the Alliance for Continuing Education and the Health Professions Annual Meeting in New Orleans in February. Lovely.
And I did also want to say that you're all the things that you said you didn't want to talk about because they might be boring are not boring at all because I've been on your website. I've read some of the backstory.
I've seen you perform on YouTube. Perform is the wrong word. You know what I mean.
But we all have a bit of performance. None of this is boring.
Well, thank you. Thank you. Yeah, right.
Yeah, I'm very passionate about the work that I do and in all the different ways that I do it.
And I think it makes it interesting for myself and of course, anyone that comes into contact with me.
[5:18] So given that that's the case, you do have a broad background.
[5:22] You've done a lot of different things that are all really kind of interesting and relevant.
And I always really appreciate hearing how all the different threads of people's professional journeys connect at a certain point and really create a sort of fascinating creative knot that anchors them to the work that they're kind of currently doing.
So how do you think all those threads inform what you're doing now and the approach that you're taking to all the things you talked about, learning strategy and measurements and evaluation?
[5:58] So this is an interesting question, and it's taken me just time to see the thread connecting all of the things.
Because if you do look at my LinkedIn profile, you'll be like, what the heck was she thinking?
She went from teaching English as a foreign language to marketing to human sexuality education to nonprofit to real estate investing, and the story goes on and on.
The thread, I can tell you, Alex, is that I have always had to justify what I'm doing and why in the various roles that I've played.
And that thinking and the necessity of having to justify my work began early, early in my career, early 20s into my first jobs right out of college.
So it really had a chance to shape my thinking leading up to today.
[6:49] And so the justification, I wrote some examples down just to give some clarity around that.
So why justifying our work matters? Well, some examples of that would be calculating a preliminary return on an investment or return on expectations for work that you're doing or adopting or investing in technology tools or hiring people to support you.
Essentially, the justification process gives us really clear strategy on what we're doing, and it helps us to envision what does success look like.
And through that envisioning process, we get some ideas of metrics on the front end and some of that kind of data we will be looking to collect to know if we were successful.
And that justification process involves all of those things.
So, for example, when I worked for the nonprofit sector, we had to write grant proposals and the learning and development department was very involved in that because we were part of the delivery of those grant outcomes.
And so a bunch of us would sit in a room and we'd talk about what are we doing with this money? Why are we applying for the grant? What are our deliverables?
And because you can imagine with grants, they want to know, how do you plan on telling us that you were successful? Right.
So that kind of thinking is inherent in the world of nonprofit, even government based learning and development initiatives.
[8:09] The other piece of the puzzle in the way that justification showed up for me was when I worked in public health.
So we were grant funded, but we were also government funded and we also published our work.
And so we had to do what's called evidence based curricula. So we had to look at the evidence of how it has come before us in terms of the work in educational public health initiatives, how were they successful, what contributed to their success, and how are we going to lean into what's worked and what hasn't worked to then inform the process.
Process, and then we have to justify it by writing it up, creating a proposal before we could even get approval to move forward.
So again, a lot of the work was always done on the front end, well in advance of delivering something.
And then academic journal writing, I've done quite a bit of writing in peer-reviewed journals, and they all ask you for your methodology.
Methodology is part of a process of justification, and the methodology has to be clear and concise and make sense for the project and the outcomes that you're seeking.
[9:13] My very first job out of college, my very, very first real big girl pants job, I worked for a company that was incredibly data-driven.
I had no idea at the time that they were data-driven, but now with all of the years that have gone by, I can honestly say they use Salesforce well before any other company used Salesforce.
They had codes of conduct for how you input your data.
We had to pull reports, and we were constantly working in our one source.
It was like our one source of truth was inside of Salesforce in terms of our customer base and our initiatives and everything was there.
And now I can say that that organization so many years ago, this was back in 2006 or 2007, that this particular organization was really mature in how it used data and they were very successful.
Successful but that was because of their data-driven mindset so these are all just examples of being data-driven and having to justify our work which includes you know needs analysis evidence-based collecting data on the front end what are we doing and why that's really set me up for success and just now intuitively thinking like that with any kind of project that I approach.
[10:26] No that's great and I you know everything that you just shared here will resonate with podcast listeners here because continuing medical education and continuing education on the accredited side, and there's accredited and non-accredited, but we're talking about accredited education, which obviously has a very kind of clear compliance framework around it.
This is a very evidence-based, data-driven, outcomes-driven field for sure.
And also people are always talking about value.
How can we show value internally and also externally to our learners, to our supporters, to the people who stand to benefit from the work that we're doing in CME and CE.
And I think it's also a good reminder that a lot of what we do in CME really has strong parallels with what you find in public health.
Absolutely. And, you know, there are some links between CME and public health, but actually the way the fields operate are very similar.
So, you know, I think that's a really kind of interesting analogy or set of analogies that you shared in relation to your own work.
[11:39] If we're thinking about, so, you know, we're talking about all these personal threads that have come together in your professional life and, and you mentioned, you know, they form an intuitive framework for you now.
Can you share a little bit about, you know, when we're talking about outcomes and evaluation, how does that framework kind of pan out in your mind?
You know, what's, you know, how do you describe that? Is there a way of, is there a model?
Is there a framework for talking about what you mean when you're talking about outcomes comes in evaluation. That was a very long question.
It was. To paraphrase your question, I'll say, how do we know that we're doing evaluation?
And how do we know that we're doing the right work to get outcomes?
And so I think about the answer in terms of three checkboxes.
If we can check these boxes, then we know we're on the right path or moving in the right direction toward truly truly valuable outcome and evaluative work.
And so those three things are, one, that if we've done evaluation in the right way, at the end of the evaluation, we should have proof that.
[12:47] That we were successful or not, and why or why not, when it comes to whatever we were doing. That could be a learning intervention.
It could even literally be a small intervention like, hey, we're going to pay people more and hope to see at the end that they stay with us longer.
Whatever the intervention or initiative is, if we're evaluating it successfully or properly, we know if we were successful or not, and we have hard, trustworthy proof around that. that.
That's one. The other is that we've got data to improve our work.
So if you've got a program initiative and you had a goal, well, you either succeeded or oftentimes you'll have succeeded in some degree.
Probably you've got 80% at best of success. I don't think any of us ever see 100% success unless we've been iterating on a product or program over time.
But we should have good feedback to help us improve.
So if you don't have any information or insight as a result of your evaluation to help you improve, you look at the data and you're like, I don't know what to do with this. This doesn't help me.
Yeah, your evaluation probably wasn't on track.
[13:57] And then the third thing is the ability to make decisions, operational decisions, growth decisions, future directions for product or program development.
We should have data and insights from our evaluation work that help us to make key operational and business decisions.
And if we don't, if we look at our data, and again, we're like, I don't, this doesn't help me to make a key decision, then we probably need to reevaluate our evaluation strategies.
And so what do robust evaluation strategies look like?
So to give you, you know, a flavor of, you know, the approach in the CMECE field.
[14:41] You know, one of the models, one of the frameworks for evaluating outcomes is called the Moore's seven-level framework.
And that kind of stretches, I don't know if you're familiar with this, but it stretches from, yeah, participation, satisfaction, knowledge, competence, performance, patient outcomes, community outcomes.
And a lot of the evaluation strategies do tend to kind of cluster around knowledge and competence.
And so, you know, they're really kind of focusing on, you know assessment as much as anything else looking at learning lift and you know maybe behavior change but in the work that you're doing with non-profits and public health organizations you know what are some of the evaluation strategies that you see your clients using or that you are recommending to your clients that can really provide the things you've just described in terms of those insights for not only whether something worked, but why it worked, because I think this is a kind of crucial missing piece for a lot of evaluation strategies. Yeah, great question.
And I will say, Alex, that in my research, I've encountered at least 40 different frameworks, models, tools, or resources in the world of measurement and evaluation in that are sort of in and around education. So that could be higher education models. It could be CME, this model that you just described.
[16:05] It could be leadership development. It could be learning and development in the corporate world.
You get the idea. There's a ton of models.
Yeah. And then there's, of course, human resources and blah, blah, blah. I could go on for ages.
But we have these like discipline specific models. And you're right.
At the end of the day, What all those models have in common is that they're essentially looking at, did we make changes in people's thinking, in their knowledge base, and of course, in critical actions and behaviors that we wanted them to do with their newly acquired knowledge.
Knowledge and if i look at at a really base level and this is just this is just the research sort of mathematical side of things like like forget those 40 models at the end of the day if you just look at the mathematics of it which is a formula we're looking at a couple of variables we're looking at inputs we're looking at outputs we're looking at outcomes results and impact and all of those models use some version of those core formulas, and then they format them in a different way and help us with some key thing that could be industry-specific or whatever.
[17:17] But truly, what I think is really helpful is that we strip away all the models that we've been taught and we go to the basics.
And I can say, in learning and development, most people are familiar with the Kirkpatrick model or the return on investment model.
There's nothing wrong with those models, But if we don't like understand at a deep level, like the basic level, what are what are those models trying to do?
They're trying to combine in a meaningful way the relationship between inputs, outputs, outcomes, et cetera, et cetera.
And so we just need to create a strong chain of evidence among all of those things.
[17:56] Once you do that, then you can calculate return on investment.
Well, how much did it cost for all the inputs that we saw, all the activities that people did? What was the cost of that?
And then we have our outcomes and results and we have our impact.
Did we get the returns based upon the financial inputs that we invested?
Like you can calculate ROI with the combination of those variables.
You can calculate behavior change. You can calculate knowledge, growth, et cetera. So at the end of the day, being able to line up all of those variables in a hypothesis and say, this is what we're doing and why.
I have an impact hypothesis that I write about in my book and I teach in workshops, and it's like a super stripped down basic formula that helps us to strategically align our variables before we do anything else.
So kind of going back to like justifying your work when you do your math equations, like before we can turn in our homework to our professor, we want to make sure we're showing our work so that the professor understands that we truly know what we're doing and why. The same concept.
So, you know, what are what's your program supposed to do in terms of like, what are the activities that people have to do to get the outcomes?
And how are you going to calculate the percent of completed activities?
[19:17] Because we don't get to go any further down the chain of evidence if people aren't doing the work.
Well, you got to solve for that first. And that's probably an engagement problem.
And that's a whole other conversation.
[19:27] But you're lining up these, I think of them as dominoes. Each domino has to be knocked over in order for future dominoes to be knocked over.
So of course, you have to have, you know, you can calculate inputs, but I don't recommend that if you're not trying to calculate an ROI or an efficiency outcome.
We don't always care about that. Sometimes we do, sometimes we don't.
And you can always look back at that too. It's an easy thing to calculate in retrospect.
But ultimately, what are the activities that people need to do to get the knowledge and behavior change outcomes.
Well, then what are those knowledge and behavior change outcomes?
Those would be like the immediate sort of outputs or outcomes, depending on what the thing you're looking at and get really getting really clear on that.
And then what are those immediate term, short term and long term outcomes that we seek because people have this knowledge and they're practicing this new performance?
What does that do for our community?
What does that do for our business? And it's a literal like you could.
[20:24] Do this in an hour. You could get the right people in a room and you can go, all right, y'all, what are our dominoes here?
What are we expecting people to do? What changes do we expect to see?
And why do these changes matter?
Those are all of your hypotheses you line up.
And then you ask yourself, what data do we need to collect to prove if these things are happening or not? And that's where the real fun begins.
And that's where some of these other models can be super, super, super helpful.
But without that high level strategy, kind of aligning and advance all of those key variables, it's super easy to get lost in the weeds of any measurement model, because they have their own agenda and solution.
They're not right or wrong. But without that strategy clearly outlined, it's hard to leverage the tactics recommended in all of the various models that are out there.
[21:11] Yeah, that totally makes sense. Do you see a difference between output and outcomes?
Yeah. So output would be like, we have these activities that we wanted people to do, and then they do them.
So common outputs of immediate classroom or formal learning would be like presentations, would be like role plays, or maybe preliminary outcomes of the activities that they do inside of the classroom.
So like teaching somebody how to use a database, base.
They're probably going to input some data in the beginning and the data that's been input into the system. Well, that's an output.
The outcomes are what become possible because those outputs took place.
And so, you know, some examples would be if we see that people's behaviors are starting to preliminarily change and we can see that inside of a learning program, the immediate outcome is, well, what does that do for the business?
And so the outputs and outcomes can often be confused.
And really, the output is more at that activity level, what are people doing, the outcome is what becomes possible when people do those things.
[22:21] Yeah, no, that's a that's a really clear explanation. I appreciate that.
And we're talking about, of course, we're talking about outcomes.
And you've mentioned businesses and organizations a couple of times, but in the learning and development world, you know, often we're we're thinking about outcomes in terms of knowledge or competence, sometimes performance.
[22:38] Performance. But what about process?
You know, how important is process to evaluation models?
And what are some of the ways that you see your clients? Or do you recommend people collect process data?
And then and then what do we do? So, so I think there's a couple of ways to answer your question.
But what I imagine you're asking are like, what are the processes we should be be following when it comes to doing some of the evaluative work?
Or how do we evaluate if processes are being employed as part of learning?
I just want to clarify that I understand the direction of your question.
[23:15] Yeah, that's a great question because actually I'm thinking process in terms of process evaluation.
So at the beginning of our conversation, you talked about when we're evaluating, we're talking about not only the outcomes, but why those outcomes occurred.
So in public health evaluation, for instance, a process evaluation is trying to kind of tease out all those factors that potentially contributed to the outcomes that we're seeing. So I'm thinking about it in those terms.
Yeah. Yeah. And to be honest, I feel like to answer it super simply is just to lean into that chain of evidence thinking and making sure that that chain of evidence makes sense.
And that's kind of a double approach. It's like, are we using the right process in terms of going into our evaluation strategy?
And then we can evaluate if the process that we used in terms of what data that we collected and that chain of evidence?
Did we see those dominoes get knocked over by looking at the data that we collected?
I hope that answers your question. It might not be exactly what you were looking for, but at the end of the day, the small, simple strategies to help us get started can often be more effective than getting lost in the weeds of all the ways in which we could methodologically be doing evaluation.
[24:34] And I have some examples of that because I know you asked me to reflect on summative, informative assessments, and I have so much to say about that.
So let's dig into that. Yeah. Well, I wanted to share, I was thinking about processes in like.
[24:48] Let's step aside for a second on the methodologies, because there are millions of them, and they are unique to industries.
But at the end of the day, there's basic exercises, if you will, they can be called processes, which is why I liked that term, like process is kind of neutral, like what should we be following?
And one of the things that I think is really useful, so in addition to this chain of evidence process thinking, if you will, another process is answering the question, is what your program intended to do, is it intended to facilitate engagement?
So is success really measured by people engaging in it or is change?
Are you intending to facilitate change on the other side of that learning experience, that program or that initiative?
And I love this categorical process of like, what camp does your program fall into?
Because it's easy to get lost in the weeds on like all these different methodologies.
But if you just simplify it, like, are we successful because we saw an increase in engagement or we saw engagement just fundamentally great?
Or do we need to see something more than that engagement going beyond that into change?
And then building the practice of what do indicators of change look like?
[26:07] So the process, in addition to the chain of evidence process, that's simple and easy, and we should employ at the start of our.
[26:18] Any kind of project we begin. The other process is the categorical identification of, is your program designed to just get people to engage in it?
And is that the value in and of itself is just getting people to engage?
Or is the value in the change that's facilitated on the other side of your program?
And to build the muscle of looking at what data artifacts facts or what indicators might tell me if that change or if that engagement took place.
And that's just a really great thing to practice in our everyday lives is like, oh, I'm trying to lose weight or I want to get healthier.
[26:59] What are the indicators that I have progressed toward my health goals?
And just literally practicing that thinking, oh, well, maybe I would lose weight, but maybe if if I'm lifting weights, I might actually gain weight.
And if my indicator of health was that I would be losing weight, well, that's a bad indicator.
[27:21] What are other indicators that might also tell me if I'm increasing my health?
Well, I might actually measure my waist or I might measure certain areas of my body that I would like to see decrease in their actual size.
Or one of my favorites is I have a pair of jeans that is my indicator of how fit I am.
And if I can fit into to those jeans comfortably, well, then I feel like I'm maintaining my health goals.
If my jeans are a little too tight, well, I might have to spend some more time at the gym.
So these are just like three different indicators and they're all completely different that might tell us, are we moving in the right direction with our health goals?
So the same is true when it comes to change. What's the change that you want to see and brainstorming? What are all the indicators that might tell me if that change is taking place.
And then where do I go to get the data to help me monitor those indicators?
And then we can select the indicators that make the most sense with regard to how much time it takes to get the data, do we have access to it, etc, etc.
So from my perspective of process, that thinking and building that muscle is incredibly helpful when when we're trying to boost our measurement and evaluation skills and processes.
[28:40] No, I love that. And I, I really appreciate the granularity of the description about indicators, because I think this is an area that can be a little bit challenging for people.
And you, you kind of hinted at this as well, in terms of getting into that mindset, that habit of thinking in terms of indicators.
Do you have recommendations for people on how they can start to build that indicator muscle?
I always go to your personal life. So.
[29:11] We have institutional barriers in our workplace that often make it feel sometimes nearly impossible if we don't have access to data and we're frustrated or whatever.
We have these energetic barriers sometimes in the workplace around working with data and measurement.
So if you want to build the muscle and the workplace is not the ideal space to start start building that muscle, then start building it in a space that has less barriers.
And for me, that's my personal life, specifically with a goal or an initiative that's really important to me.
And I like to use the example of health and weight loss because many of us resonate with that.
It can be something like you're trying to buy a new house and you want to save some money and change how you're spending and managing money in order to purchase something important to you.
There are ways that we can, what indicators would tell us that we're moving in the right direction with our money spending habits?
Do I have more savings at the end of the month? Do I find cash in my pockets when I do laundry more so than I did last month, right?
There's all kinds of indicators, but the muscle is brainstorming, what are we trying to do?
And the indicators are just telling us, are we moving in the right direction?
And that's why I love them is because it's something to track and monitor over time.
[30:37] And once we get to a place where we consistently see those indicators are moving in the right direction, we don't have to spend as much energy monitoring those programs anymore, because at that point, they're kind of doing what they're supposed to be doing until we have to reevaluate them for some other reason.
So I appreciate that perspective. perspective. And I think, yeah, no, I think it's a great perspective.
One question I have there is, and I'm wondering what your perspective on this is.
[31:04] Is, you know, one of the key pieces of thinking about and brainstorming indicators is behaviors, because all the things that you've just kind of described are behaviors.
And I think for some people, it can be really challenging to break down the behaviors that contribute to change.
[31:24] Is that something you see in the clients that you work with?
Is this a barrier to brainstorming potential indicators for change?
Yeah, I think 100%. Or is it just me? Nope. Yes.
And it's even more challenging in soft skill training and leadership development, which is a lot of the work that many of us are doing in one way or another.
And we often, I've seen on social media that it's like, it's not worth it to try and measure outcomes of soft skill or leadership training because it's too subjective.
And that tells me that we haven't done the strategic thinking on the front end to know, well, why are we investing in this leadership development training in the first place?
And it's a fair answer to say, we just want to offer professional development.
That's it. And if your goal is just for any course or program that you're offering, if you just want to offer it and let people engage in it as they feel interested and excited, well, that's great.
And I think that's learning for the sake of learning and there's value in that.
[32:22] But I would argue that leadership development, because it's very costly, is generally we're trying to see some kind of change.
And that change might be that we hire more people, excuse me, we promote more people from within versus needing to look externally for talent because we haven't cultivated talent inside of our own organizations.
So why are we investing in the leadership development training in the first place? And is there a specific change that we want to see on the other side of that? And what is that change?
And if we can't clarify what that looks like, then it gets really difficult to get down to that micro behavior.
[33:03] You know, observable changes at that level, if we don't start with what's the change?
Well, and then how do we know that change has happened? And that becomes an easy brainstorm.
How do we know that we have more people who are ready for promotions inside of our organization?
Well, what does that look like? What are those indicators? So having a clear outcome of whatever it is that we're doing.
Another great example is communication training. We see this all the time.
And we also know that there's a lot of conflict inside of organizations and teams.
So one possible change that comes about from communication training is a decrease in conflict.
Well, if once we have clarity on that change, then we can go to a team meeting and say, well, what are all the ways that we might see conflict look different?
What are those indicators that conflict has reduced?
And this is where multiple perspectives on an answer to this become imperative because we don't all think the same way.
And indicators of change can look a lot of different ways.
So having people from a diverse subset of the organization reflecting on this, especially if they're going to be benefactors themselves of the outcomes of the program, they should have input into what those potential indicators of change could look like.
And then we figure out what are the indicators that are the most realistic for us to track. And that's how we get to our metrics.
[34:30] What are you seeing as some of the emerging trends or things that professionals in learning and development will need to pay attention to?
So, of course, you would expect my answer to this, but how are we able to use technology, especially things like generative AI and chat GPT, to make our role, whether it's an instructional design or measurement and evaluation, easier?
And ultimately, for the purposes of increasing the quality of our work.
Yes, it's great to get time back.
But we want to also see that being more strategic with technology use is improving the quality and the user experience and then the outcomes of the work that we're doing.
And so one of the things I included in my book is the latest study on what are people currently doing in terms of using chat GPT and other generative AI technology.
And so people are definitely using AI to create full courses, to create videos, to do transcriptions. All these things are intuitive.
[35:36] What they're not doing is using it to help them measure and evaluate.
And as just one example, I literally just did this three days ago.
I wanted to test ChatGPT, just the free version, not the paid version, to see what it can do in terms of helping me save time creating a measurement or an evaluation process.
So I use the example of communication skills and I put in the chat GPT, what evaluation options are available to evaluate growth in communication skills?
And chat GPT came back with like eight different options like psychometric exams, observations, self-assessments, surveys, blah, blah, blah. You get the idea.
And then I was like, okay, that's interesting, but it still makes me do a lot of the work.
So I'm like, okay, let me see if I can make this more complex.
So I'm like, okay, if I wanted to do a psychometric assessment for communication.
So I said, okay, what psychometric assessments are available to help me evaluate growth in active listening?
So I had to get more specific, again, based on the change that you want to see.
[36:44] Asking about communication skills was too broad, but growth in active listening helped help chat GPT to then come back with seven options of existing psychometric evaluations that we could purchase off the shelf that would show changes in active listening.
So if you wanted to pay for one of those and use that to evaluate growth, that was an option.
I'm like, okay, let's go one more layer complex.
[37:09] Let's ask it if it can help us to create our own rubric if we we wanted to do observations.
So I said, I'd like to use observation of a role play activity.
Can you make a rubric that helps me to observe growth in active listening skills?
And it was, it came back with a very robust rubric that had six different criteria of active listening.
And then it had four or five different points on a scale of excellent to poor with examples from a micro microbehavior level of what you would see somebody doing at the excellent to poor scale for all six of those criterion.
And I'm like, wow, that's pretty impressive.
[37:54] So I would like to see that we take advantage of some of this technology.
But Alex, none of that was possible. I couldn't leverage chat GPT if I didn't know what was the specific growth that I wanted to see, aka active listening in this example exactly and what were the methods that i might select to use relevant to the delivery and the sort of structure of the program so maybe a psychometric assessment is relevant maybe it's not and so we have to know those things in order to be able to leverage technology so yes trends are in the technological side of things but in order to leverage technology We've got to build that muscle of knowing our strategy in advance, justifying what we're doing, creating those indicators of change such that we can ask things like chat GPT to get ideas or help us to craft rubrics or other types of tools to do the hard work of evaluation.
[38:54] Yeah, no, I completely agree with you there. You know, we see that in the writing world and the content creation world as well.
And I'm talking about medical writing and science writing.
You have to be really clear about what your parameters are.
You have to be able to prompt in a very specific and contextualized way in order to kind of, you know, provide the right information for generative AI.
And of course, ChatGPT isn't the only tool out there now. And so your expertise is still front and center.
[39:26] And, and that kind of takes us back to, you know, learning and development and the sorts of things that people need to be skilled and schooled in, in order to handle what's in front of them and handle, in this case, technology. technology.
This has been a whirlwind of a conversation.
And of course, as ever, you know, I think we probably have a lot more to talk about.
But in the meantime, where can people find you? So I am at dralaina.com.
That is my website, my name, you probably have it in the show notes, but A-L-A-I-N-A.
I am very active on LinkedIn. In fact, I do two LinkedIn audio live sort of Q&A sessions where we dig into some of these measurement mysteries and evaluation strategies.
So feel free to tune into those.
And I have a weekly newsletter that, Alex, you are a faithful subscriber of, thank you, where we talk about some of the latest tools and resources and just, you know, ways of thinking about measurement to make it easier on us as we continue to peel that onion of getting better and better at measuring our outcomes.
And thank you so much for sharing your wisdom and insights with listeners of Bright Medicine. You're very welcome.
[40:51] Effective evaluation in any field requires a balance of trustworthy evidence, improvement data, and operational insights.
So here are three takeaways I heard from Elena to help strike that balance.
[41:05] First, consider creating an impact hypothesis by lining up dominoes.
Start with the change you intend to make, then trace back through outcomes, outputs and inputs.
Visualise this sequence to clarify your strategy and prepare to measure each stage.
Second, develop your indicator muscle by applying evaluation strategies to personal goals.
Choose a goal, define your indicators of success and track them over time. time.
This type of practice can help build your skills in a lower stakes environment, and prepare you for larger, more complex projects.
[41:44] And while AI is commonly used for course creation, it's not as widely used for measurement and evaluation purposes.
But tools like ChatGPT can assist in creating evaluation processes and rubrics, but it's essential to know the specific growth and methods relevant to the the program you're focused on.
We're back on Monday with Monday Mentor, and on February the 21st, author Ben Lewin joins me to talk about his new book, Inside Science, which challenges the traditional view of science as an objective, self-correcting process.
In the meantime, hop on over to the blog on my website for more resources on CME, and don't miss out on Right Medicine episodes.
Subscribe to the podcast on your favorite listening platform.
There's a link in the show notes. Till next time, stay curious and keep learning.
Dr. Alaina Szlachta, Learning Architect at By Design Development Solutions, improves the results of personal and professional development programs through data enablement. She hosts a community of practice, Measurement Made Easy, to explore simpler solutions for showing program outcomes and impact. She’s also writing an ATD Press book, "Measurement and Evaluation on a Shoestring," written to make M&E easier and more accessible.